
The convex relaxation for matrix rank that measures how compact the representations are, which is widely applied in machine learning. The sum of singular values is the nuclear norm, i.e., We compare the accumulative ratios of squared top r singular values over total squared singular values of the unfolded feature maps ( i.e., R C × H W) before and after passing through the attention module, illustrated in Fig.
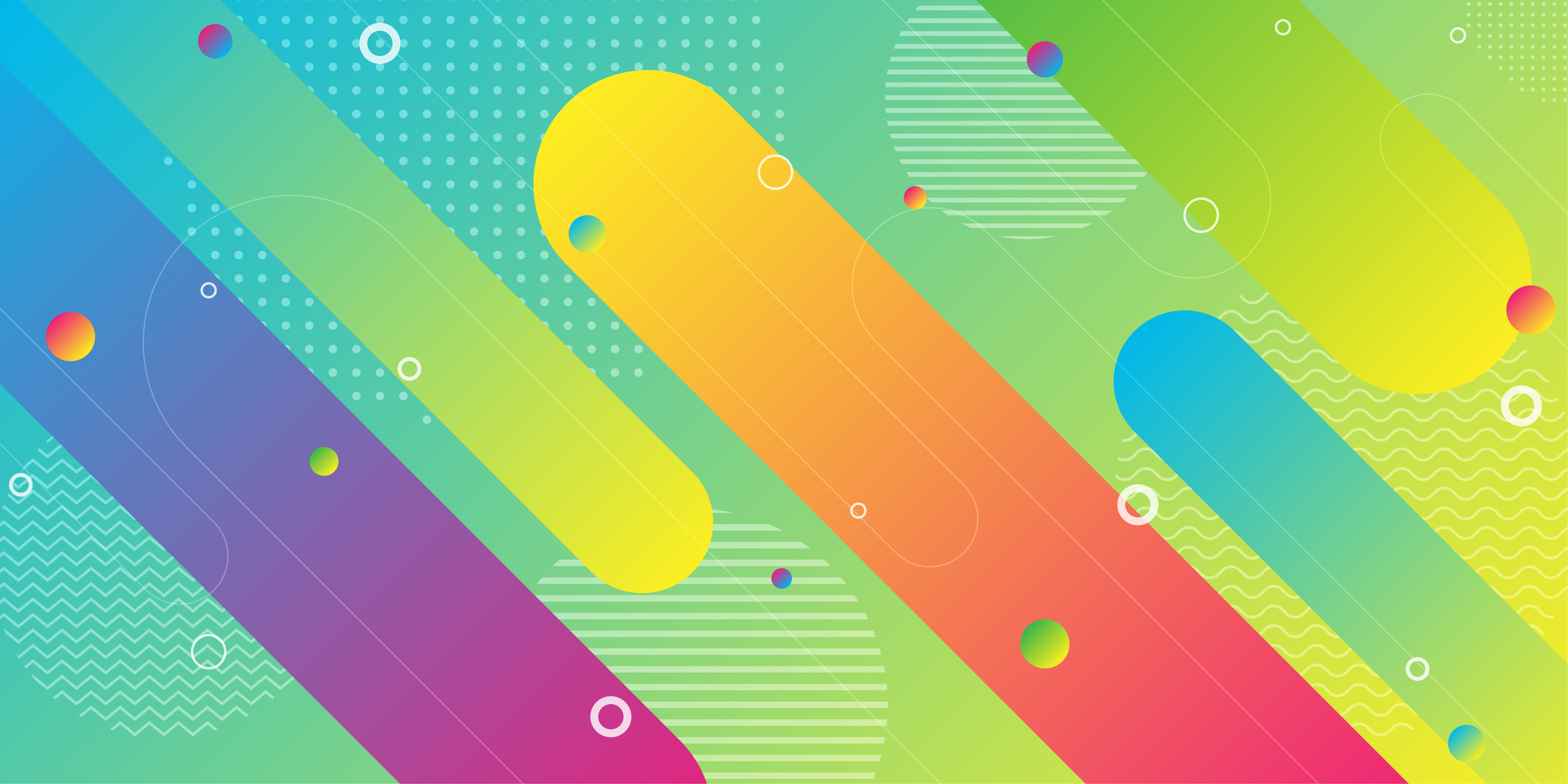
The ratios of feature maps before and after the attention module in SCFT and SGA are displayed.įurthermore, we explore the superiority of SGA over SCFT in terms of rescaling spectrum concentration of the representations. Quantitative comparison with different methods.Īccumulative ratio of the squared top r singular values over total squared singular values in feature maps. 7, the images generated by SGA have less color-bleeding and higher color consistency in perceptual. This clear-cut improvement means that SGA produces a more realistic image with high outline preservation compared with previous methods.Īccording to Fig. With respect to our main competitor SCFT, SGA improves by 27.21% and 25.67% on average for FID and SSIM, respectively. Table 2 shows that SGA outperforms other techniques by a large margin. įor fairness, all networks use the same auto-encoder architecture and aforementioned train losses in our experiment. We compare our method with existing state-of-the-art modules include not only reference-based line-art colorization īut also image-to-image translation, i.e., SPADE, CoCosNet, UNITE and CMFT. With state-of-the-art modules in line-art colorization, our approachĭemonstrates significant improvements in Fréchet Inception Distance (FID, up This training strategy, Stop-Gradient Attention (SGA), outperforming theĪttention baseline by a large margin with better training stability. We propose a novel attention mechanism using Us to alleviate the gradient issue by preserving the dominant gradient branch Observe gradient conflict among attention branches. The instability in training, we detect the gradient flow of attention and Instance, self-supervised training protocol and GAN-based losses. Techniques would intensify the existing training difficulty of attention, for However, in the context of reference-based line-art colorization, several Information and model the long-range dependency employ the attention mechanism. Popular techniques to bridge the cross-modal Sketch, which heavily relies on the precise long-range dependency modelingīetween the sketch and reference. The color, texture, and shading are rendered based on an abstract It does not store any personal data.Reference-based line-art colorization is a challenging task in computer The cookie is set by the GDPR Cookie Consent plugin and is used to store whether or not user has consented to the use of cookies.
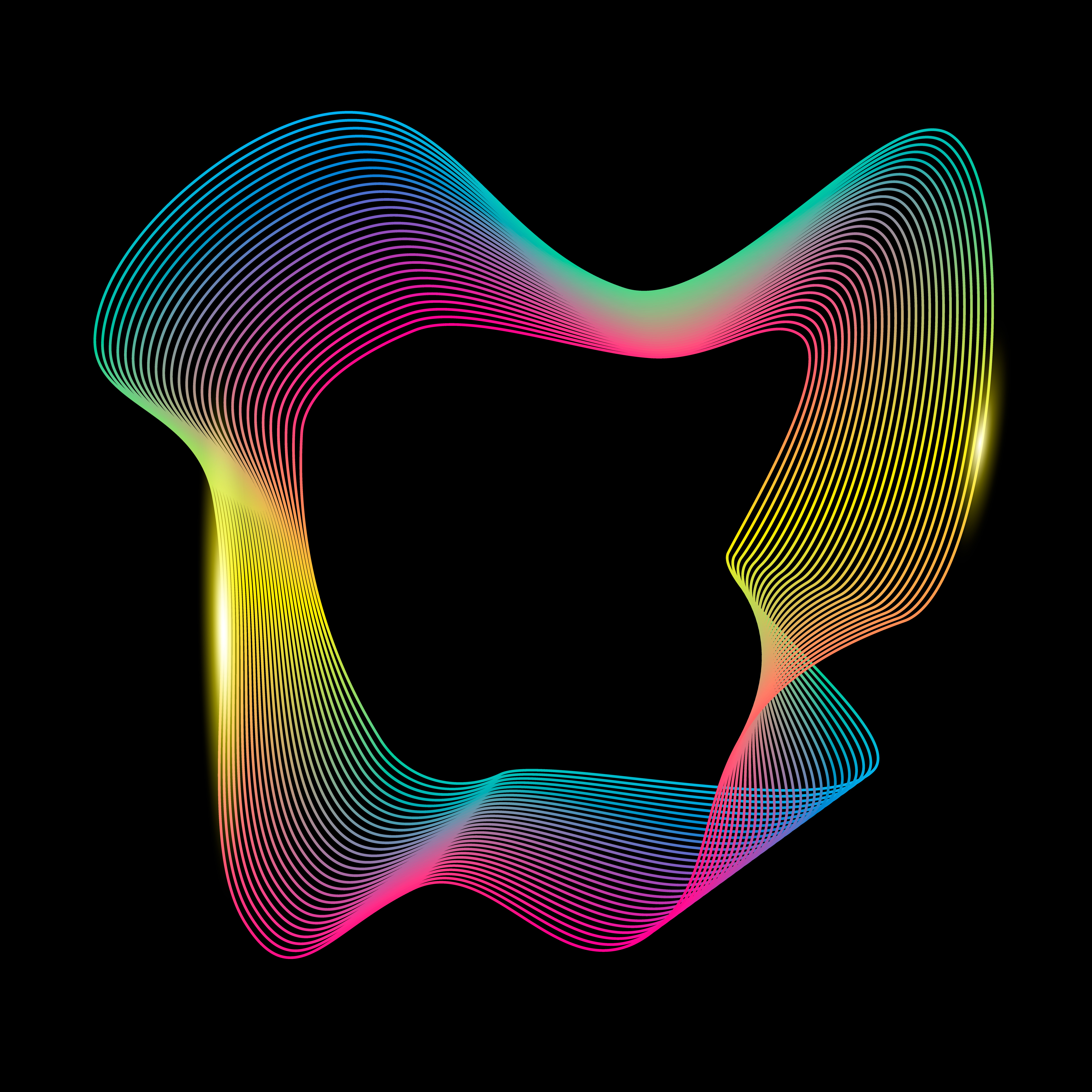
The cookie is used to store the user consent for the cookies in the category "Performance". This cookie is set by GDPR Cookie Consent plugin. The cookie is used to store the user consent for the cookies in the category "Other. The cookies is used to store the user consent for the cookies in the category "Necessary". The cookie is set by GDPR cookie consent to record the user consent for the cookies in the category "Functional". The cookie is used to store the user consent for the cookies in the category "Analytics".
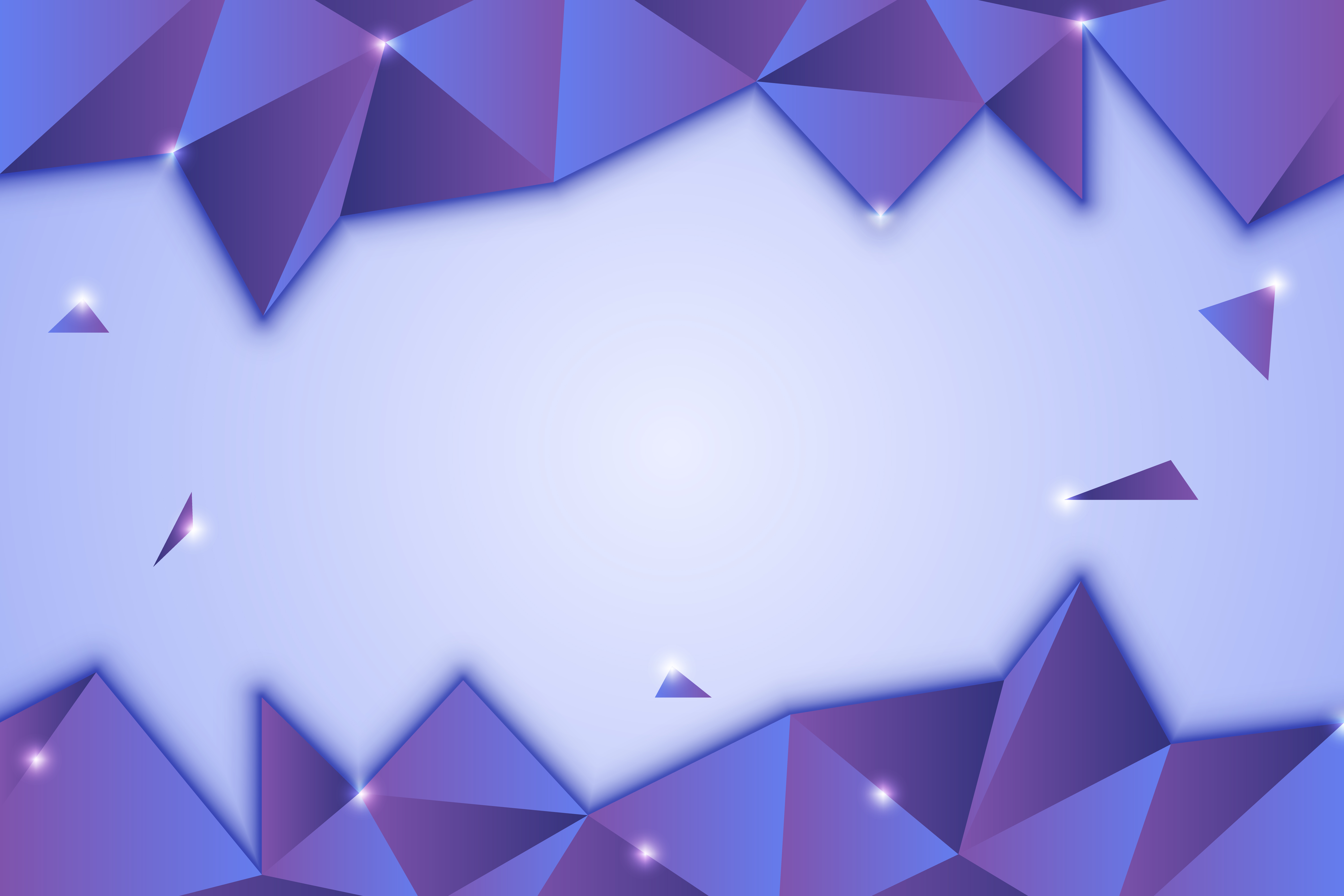
These cookies ensure basic functionalities and security features of the website, anonymously. Necessary cookies are absolutely essential for the website to function properly.

